As is tradition for AusDM we have lined up an excellent keynote speaker program. Each speaker is a well known researcher and/or practitioner in data mining and related disciplines. The keynote program provides an opportunity to hear from some of the world’s leaders on what the technology offers and where it is heading. The following keynote speakers have been confirmed:
Keynote speakers
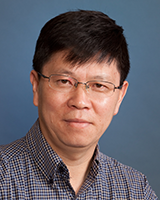
Professor Junbin Gao, The University of Sydney
Junbin Gao is Professor of Big Data Analytics at the University of Sydney Business School. Until recently his major research interest has been machine learning and its application in data science, image analysis, pattern recognition, Bayesian learning & inference, and numerical optimization etc. He is the author of 260 academic research papers and two books. His recent research has involved new machine learning algorithms for big data in business. He won two research grants in Discovery Project theme from the prestigious Australian Research Council.
Abstract – Subspace Clustering and Its Development for Manifold-valued Data
Subspace clustering is an extension of traditional clustering that seeks to find clusters in different subspaces within a dataset. The representative subspace clustering approach includes the Sparse Subspace Clustering (SSC) and the Low-Rank Representation (LLR). Both approaches rely on the so-called data self-representation to explore subspace structures among data. Theoretical analysis guarantees that the true subspace structures can be recovered even when data are contaminated by outliers. The speaker would like to report the further development of subspace clustering in the context of manifold-valued data. The focus will be given to the speaker’s recent research on clustering and dimensionality reduction tasks over a number of widely used manifolds in computer vision such as Stiefel manifolds, Grassmann manifold, and SPD manifold etc.
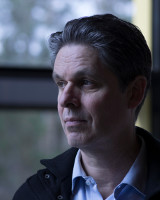
Professor Geoff Webb, Monash University
Professor Geoff Webb is Director of the Monash University Center for Data Science. He was editor in chief of the leading data mining journal, Data Mining and Knowledge Discovery, from 2005 to 2014. He has been Program Committee Chair of both the leading data mining conferences, ACM SIGKDD and IEEE ICDM, as well as General Chair of ICDM. He is a Technical Advisor to machine learning as a service startup BigML Inc and to recommender systems startup FROOMLE. He pioneered many of the key mechanisms of support-confidence association discovery in the 1980s. His OPUS search algorithm remains the state-of-the-art in rule search. He pioneered multiple research areas as diverse as black-box user modelling, interactive data analytics and statistically-sound pattern discovery. He has developed many useful machine learning algorithms that are widely deployed. His many awards include IEEE Fellow and the inaugural Eureka Prize for Excellence in Data Science (2017).
Abstract – Learning in a Dynamic and Ever-changing World
The world is dynamic – in a constant state of flux – but most learned models are static. Models learned from historical data are likely to decline in accuracy over time. I will present our recent work on how to address this serious issue that confronts many real-world applications of machine learning. Methodology: we are developing objective quantitative measures of drift and effective techniques for assessing them from sample data. Theory: we posit a strong relationship between drift rate, optimal forgetting rate and optimal bias/variance profile, with the profound implication that the fundamental nature of a learning algorithm should ideally change as drift rate changes. Techniques: we have developed the Extremely Fast Decision Tree, a statistically more efficient variant of the incremental learning workhorse, the Very Fast Decision Tree.